Most of the larger non-profit organizations we work with are scrambling to figure out how to deploy complex technologies like machine learning and “AI” in service of the social good. These include inspiring examples that range from poverty alleviation, to home fire prevention, to self-harm risk reduction. These stories have spread widely and have come to define what a data-centric organization should be doing – namely complicated data science. However, if you’re an organization thinking about how to use data better, this is not where you should start. You don’t need a data scientist, you need a data culture.
Catherine D’Ignazio and I have built the DataBasic.io tools to focus on helping people creatively build their data literacy. As more and more organizations have started using them, we’ve been pushed to think more deeply about what it means to take this approach to building a data culture. This post lays out our latest thinking abut the building a data culture, and how to overcome barriers you’re likely to run into.
The key problem we see is that organizations working for the social good don’t feel empowered to work with data in a variety of ways. This is a rank-and-file staff problem, not a data scientist problem. We’ve come to work on this in three ways: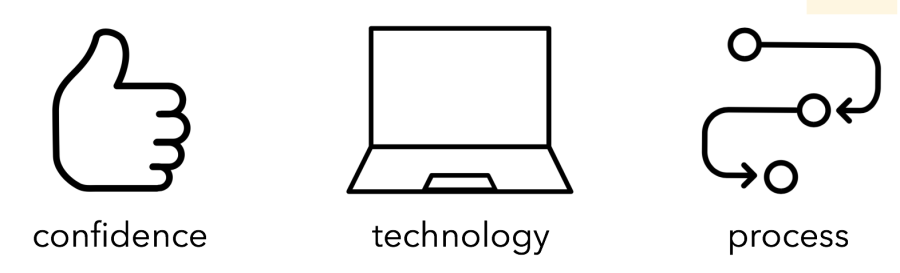
Organizations don’t feel confident that they can work with data at all, so to build a data culture we prioritize building confidence through small, focused activities. The technology that they think they need to work with data is daunting, expensive, and requires technical expertise, so our approach focuses on approaches that don’t rely on complex technology. Organizations don’t have a good process for starting to work with data, so we introduce a step-by-step approach with hands-on activities.
We’re trying to help here by creating the “Data Culture Project” – you can expect to hear more about that early next year. This gives organizations a lightweight, self-service curriculum or video-facilitated activities. We’re piloting that with 30 organizations right now, to learn from how they approach running these over three months within their organizations.
What is a “Data Culture”?
This phrase is becoming a bit of a buzz-word right now. So what does it mean? After lots of conversations, with organizations big and small, we’ve narrowed down to this list:
- Leadership prioritizes and invests in data collection, management and analysis/knowledge production.
- Leadership prioritizes creative data literacy for the whole organization, not just IT and Evaluation.
- Staff are encouraged and supported to access, combine and derive insight from the organization’s data.
- Staff recognize data when they see it. They offer creative ways to use the organization’s data to solve problems, make decisions and tell stories.
This four-part definition focuses on leadership and staff responsibility very intentionally. You need buy in across the organization to really make this work. We also focus on making sure data doesn’t get siloed into one department or another. Working with data is a core skill that can be valuable across an organization.
Why Build a Data Culture?
Why bother with building a data culture? Over the last 10 years we’ve seen a lot of data projects in our workshops and partners. These tend to cluster around three purposes.
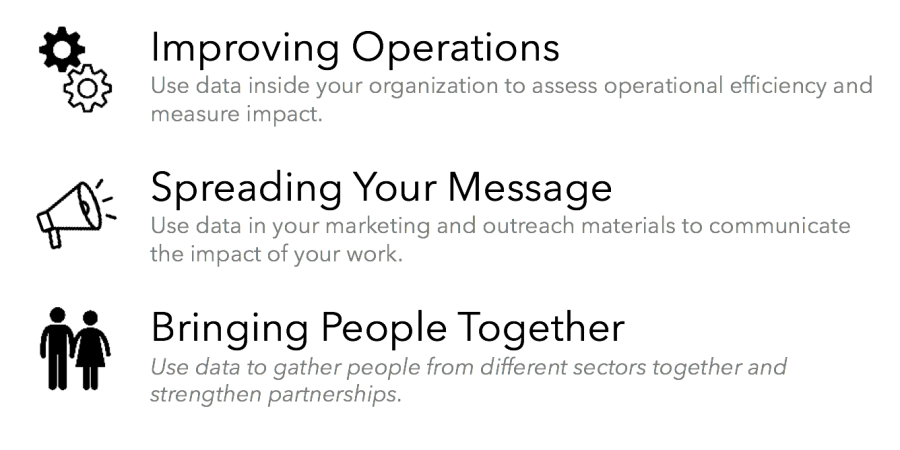
Data is most often used to improve operations; doing things like measuring delivery performance, changing how it works, and them measuring it again to see if it improved. One the last years we see more and more uses of data to spread a message, giving rise to infographics and other formats where data is used to show impact of programs. Data is less-often used to bring people together, which is the focus of my work on arts-based hands-on activities, data murals, and more. We think this third purpose is central to building a strong data culture across your organization.
Barriers to Building a Data Culture
Of course, like any organizational change, there are barriers. We’ve listed 6 that we think are useful to have in mind while thinking about any efforts you are taking to build a data culture.
Barrier #1: Confusion
Most introductions to data are confusing and overly technical.
Complicated words can alienate people that are just entering the field of working with data. Pick your words carefully to welcome them. For instance, you could introduce the idea of “correlation” by talking about “connections” between pieces of data that move together.
Piaget, the great educational psychologist, introduced us to the idea that people will absorb new information by “assimilating” it into their existing mindset, or change their mental model to “accommodate” it. If you know people’s background you can make your outreach more effective. You have to understand their existing mental models if you want to introduce new information. Your goal is not to turn everyone in the organization into data scientists. A data culture means people recognize data and are able to pinpoint new opportunities for deriving knowledge and insight from it.
Tips:
- Avoid technical jargon
- Meet people where they are
Barrier #2: Not Knowing Your Data
Sometimes you don’t even know the data you have.
At a recent workshop we were talking with a medium-sized environmental advocacy group and they lamented not having any data about participation at recent public events. I mentioned that I had seen photos on facebook, and how that was data they could use. They were surprised and had ignored this set of data, yet it contained exactly the data they wanted.
Remember that data can be qualitative or quantitative. If your development director shares photos and a headcount from your last fundraiser, that’s all data. Be creative about recognizing the data you have already.
It is hard to keep track of datasets within your organization that might be related to each other. Identify a person and a technology that can be a central clearinghouse for data. This could be as simple as a Word document with a bulleted list, or as complex as a internal data portal.
Tips:
- Keep your eyes and ears open
- Build a data catalogue, or library
Barrier #3: Organizational Silos
People will fight efforts to work across silos.
We were working with a large nonprofit to build a data culture across their organization, but they were stymied by people that thought they owned the data, and were hoarding it from others as a form of job security. The only way we found to work on it was risky – to sneakily use it and then credit its successful use to the owner retroactively. It helped, but we can do better than that.
Most organizations suffer from these silos – independent functional units that take pains to control a slice of the overall work. You have to acknowledge these walls in order to break them down.
When you have an example of a data-centric project that cuts across existing silos, hold it up as an example to success. This is an opportunity to have leadership show buy-in and backing for a cross-sectional approach to data.
Tips:
- Acknowledge your weaknesses
- Highlight successes
Barrier #4: IT-Centric Thinking
Data gets locked away in the IT department.
Over and over we hear from organizations where IT is running Tableau trainings regularly and they just can’t understand why people aren’t adopting the tool and approach. I’m like a broken record telling them that you need to separate the tool and the process – the tool training can be owned by IT, but the process training doesn’t need to be.
You need to make sure people don’t have to go to IT to pull out the latest numbers they need. Building a data culture means making sure every part of your organization can use data, for a variety of reasons.
Just because IT owns the data technology, it doesn’t mean they should own the process of creating a data culture. Building this capacity is better housed across multiple departments, or within the office of a Chief Data Scientist. That can lead to invitations to build data capacity that are more fun that just boring spreadsheet trainings.
Tips:
- Data is for everyone
- Create more invitations to work with data
Barrier #5: Irrelevance
Staff don’t connect to many high-level data dashboards.
High-level data summaries are great for leadership, but staff can’t always connect to them. You need to integrate data into their day-to-day operations. You can try ideas like mainstreaming quarterly data-reports from each department, or attaching data outcomes to program reviews. If staff don’t understand and the utility and use of data they are collecting, it just becomes boring homework they have to do. This hurts not only your data culture, but also the data quality!
Showing a number of summary of some data is great, but is just the start. Asking “so what?” is when the real culture starts to emerge. Actionable data can help you drive your organizational goal. If people can’t answer the “so what” question, then they don’t have the right data. Engage staff in figuring out why the data they collect is useful; they are best positioned to answer the “so what?” question.
Tips:
- KPIs aren’t for everyone
- Remember to ask “So What?”
Barrier #6: Boredom
Data is seen as a boring chore.
Spreadsheet-driven activities are boring to the majority of people. Use more fun activities, in novel settings, to bring a more creative approach to data. Make data sculptures in the lobby, or paint a data mural at your next retreat. These approaches create multiple pathways into learning how to work with data.
Communicating in charts and graphs is the default for presentations. However, these don’t tell a story. Encourage your organization to put the data in context, and talk about impact, but focusing on how to tell a story with your data rather than just introducing how to do Pivot Tables. People like telling stories, and get interested and engaged in hearing them.
Tips:
- Use creative data-centric activities
- Tell stories with your data
Building Your Data Culture
Each organization is different. Hopefully this high-level summary of some of our latest thinking helps inspire ideas what might work for you. In future posts we’ll dig into more concrete ways to build a data culture, the motivations behind them, and how they are working for various partner organizations we work with.
This post is based on a presentation Catherine D’Ignazio and I gave to non-profit leaders convened by the Stanford Social Innovation Review. Thanks to Catherine D’Ignazio and Ethan Zuckerman for feedback and edits.
You must be logged in to post a comment.