When people think about working with data, they usually think about the technologies that help us capture, manipulate, and make arguments with data. Over the last decade we’ve seen radical growth and innovation in the toolchains available to do this, leading to a huge increase in the number of people that have started working with data in some capacity. For data literacy learners, it is tempting to let the tool dictate the outcomes. Need to make a chart? Let the tool recommend one. The problem is that these tools don’t help you with the process of working with data. The tools won’t write your data story for you — you have to run that process yourself. Here are a couple of examples that help illuminate this gap that I see, and how my work with Catherine D’Ignazio on the Data Culture Project helps address it.
Excel doesn’t help you ask the right questions
When you’re first starting to work with a dataset on some problem, it is critical to frame a good set of questions aligned with your goals. Do you have a hypothesis that you are trying to test? Is there a specific audience you are trying to engage with the data? What assumptions do you bring to the data? Tools like Excel help you dive in to a dataset with low friction, but can’t help you identify the right questions to ask. Sometimes you need to take a step back and think about what you’re trying to achieve.
We created our “Ask Good Questions” activity to introduce this idea. You use our WTFcsv tool to browse quick visual summaries of columns in your dataset, and then brainstorm questions that could be interesting to ask it, other datasets that might help you answer those questions, and how you’d get those datasets. You don’t try to answer the questions at this initial phase, you just capture them all as potential roads to follow.
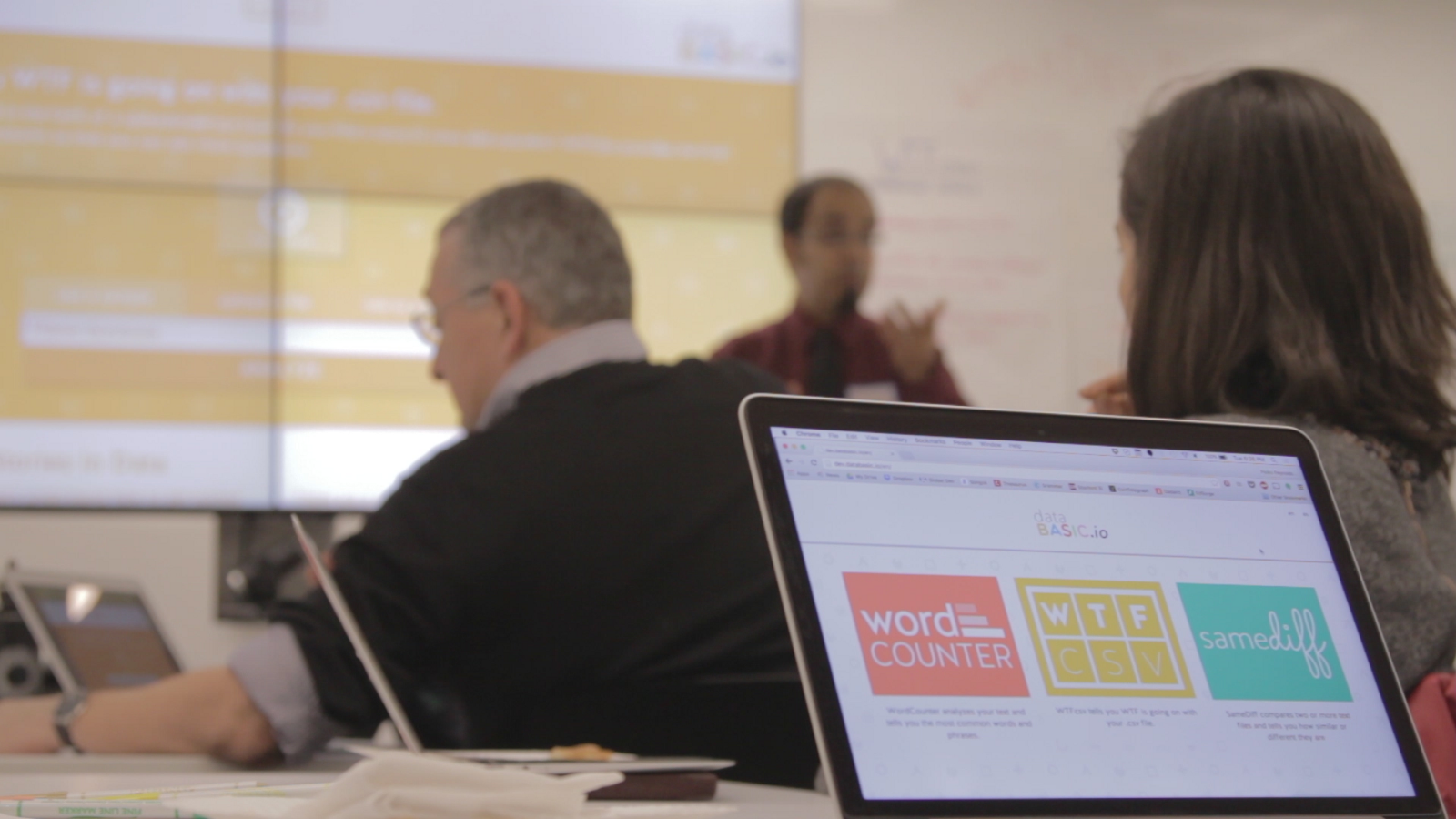
Diving into one dataset can be like putting on blinders; it limits your ability to see the possibilities outside of that single dataset. You have to take a step back to consider questions that might require you to pull in and use other datasets. Tools can’t do this for you; it is up to you to make the effort to distance yourself from where you start in order to make sure you stay on target for you goals with the data.
Tableau won’t write you a strong narrative arc
Humans think and understand the world through stories. Background context, strong characters, a clear flow from start to end — these are the key elements of story that help engage us all. Telling stories and making arguments with data is no different. Tools like Tableau can be very powerful for helping us debug the visual representation of our data stories, but they don’t scaffold the process of coming up with the narrative arc. Which characters appeal to the audience we have in mind? What resolution of our data story will drive action towards the goals we want?
Our “Data Storybook” activity helps you prototype your data story’s narrative arc. Once you’ve got a story to tell, follow our instructions to fold a big piece of paper into a small storybook. Then use comic-book or children’s-book style illustrations to tell your story over three page flips. Your book has to say “once upon a time” on the front cover, and say “the end” on the back cover. There is something magical about the page turn… it forces you to think about the beginning, middle and end of your story. This is how you work on a strong narrative arc.
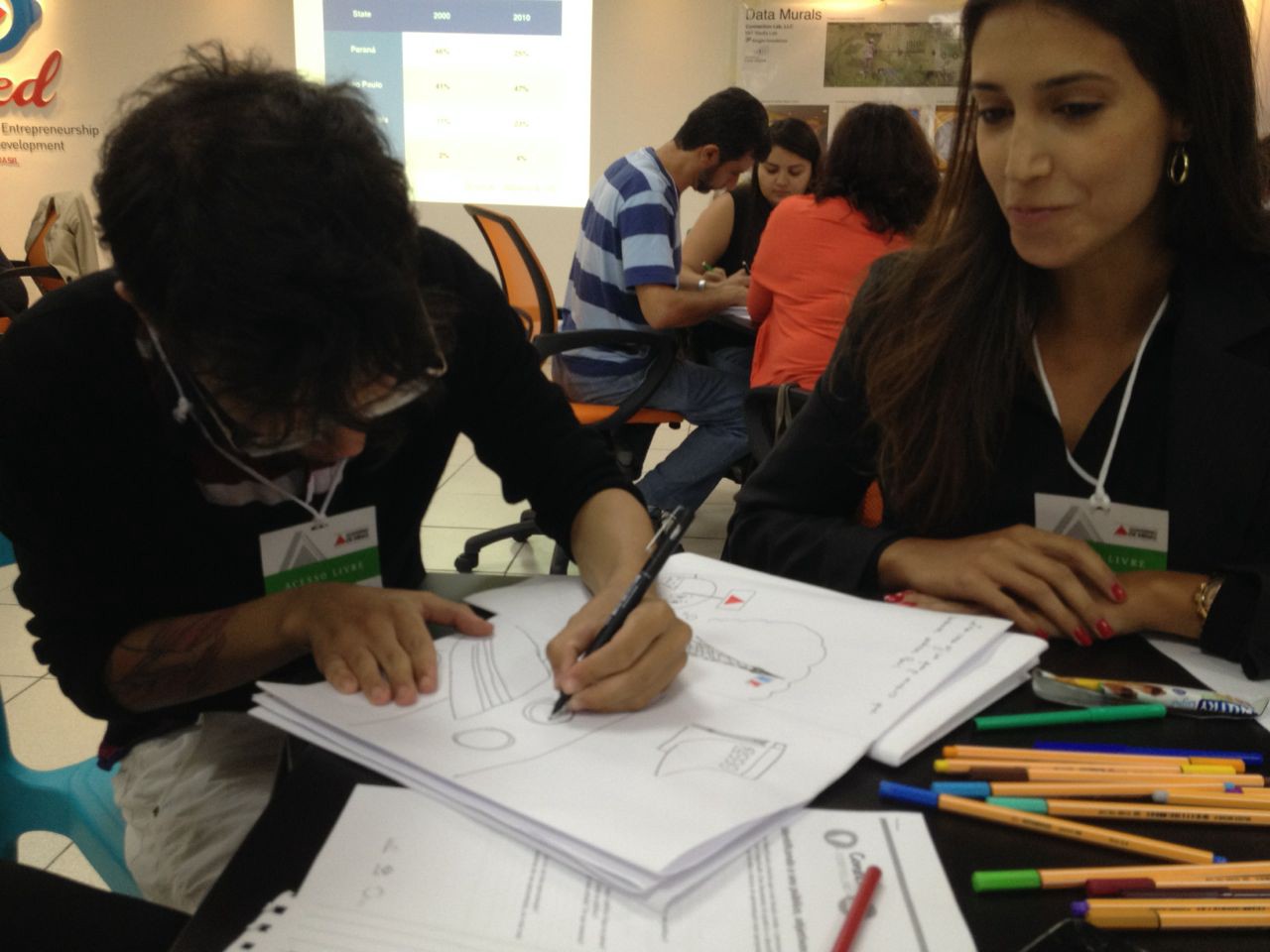
When you’re telling a data story, you have to make sure the flow is supported at each point by the data you include. Any data you include must reinforce and connect to the main narrative arc, no matter how detailed it is, otherwise you’re going to lead your intended audience astray and lose them in a confusing non-central plot-line. The tools can’t do this process for you.
R can’t help you pick the right way to tell your story
Once you’ve got a strong narrative with your data, how do you decide what format to tell it in? This decisions has to be driven by strong definitions of your audience and goals. Tools like R can’t make those connections for you; you have to consider the constraints yourself. Will readers have the visual literacy and geographic awareness that it takes to read a map visualization? Are they pre-disposed to agree or disagree with you? Are you presenting in a formal setting to an engaged audience, or on the street at a festival? These kinds of questions are critical for making an informed decision about what medium to tell your data story in.
We built our “Remix an Infographic” and “ConvinceMe” activities to help you work on this skill. The first invites you to look at the argument an infographic is making and then try to “remix it” — telling the same story as a data sculpture, creative map, personal story, or data game. Understanding the affordances of each media is critical for picking an appropriate one for your audience and goal. The second activity, ConvinceMe, helps you practice brainstorming data arguments that can drive different audiences to action. You identify stakeholders that can effect change on the system your dataset represents, invite volunteers to role play them, and then collectively try to drive them to action with creative data-informed arguments.
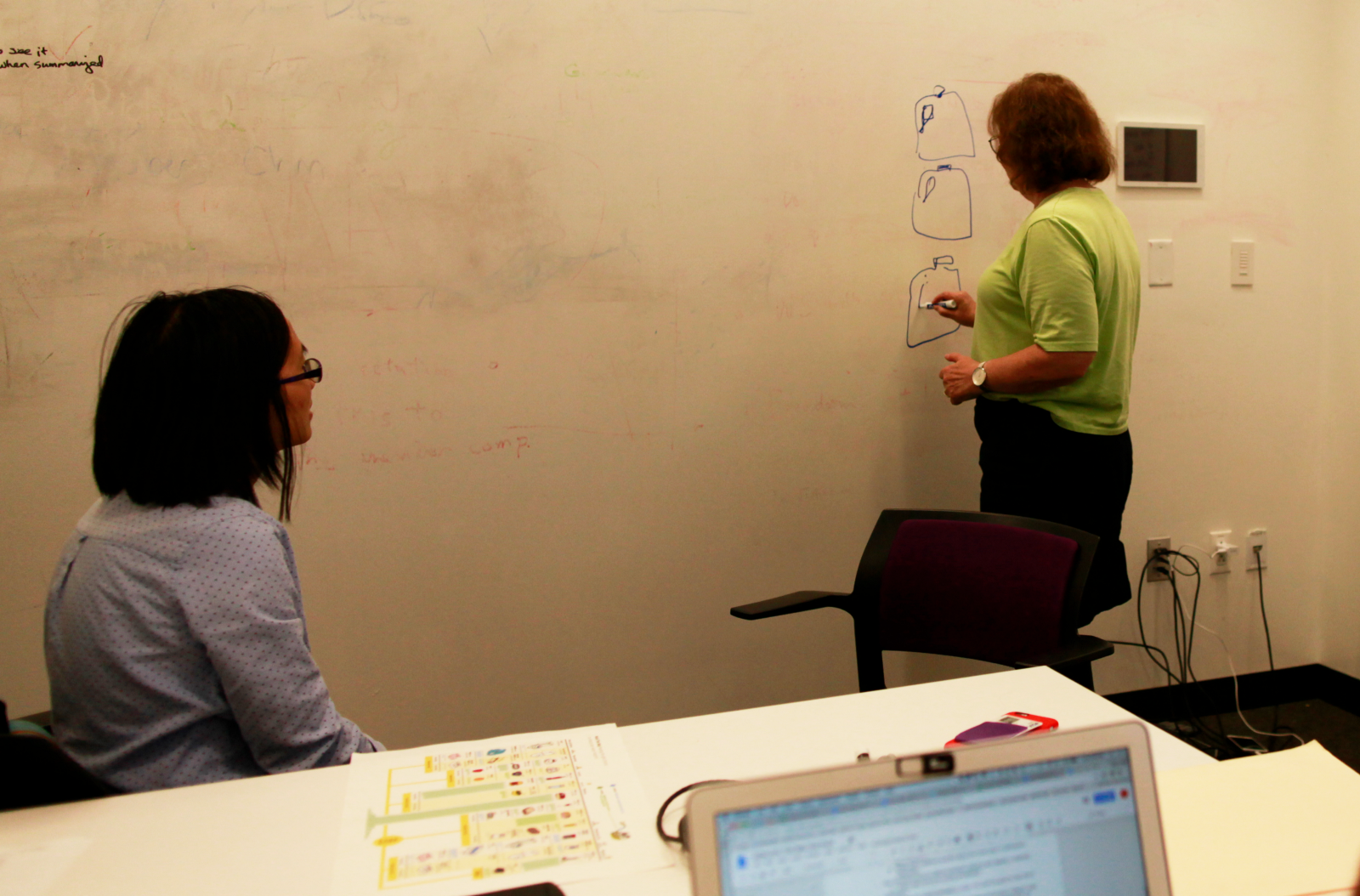
Any dataset contains a multitude of potential stories, all of which can be told in a variety of ways. The digital tools that exist don’t help you navigate that space to pick the best story, nor tell it in the more appropriate way.
Working with Data Relies on Strong Processes and Strong Tools
People think about technology as soon as you mention data. I hope these example help illustrate why I think it is so important to separate the issues of the processes and technological tools for working with data. The innovative tools can only help you so much.
Organizations around the world are using these activities I mentioned to work on the process pieces, often in parallel with their work introducing technological platforms. Non-profits, newsrooms, libraries — these are some of our many partners on the Data Culture Project.
Do you want to try these activities out in your organization or classroom? Visit http://datacultureproject.org now to see our free suites of tools for working with people in creative, arts-based ways at every point of the process of going from data to story.
You must be logged in to post a comment.